Revolutionary MST-DGCN Model Enhances Accuracy in EEG-Based Brain-Computer Interfaces
June 9, 2024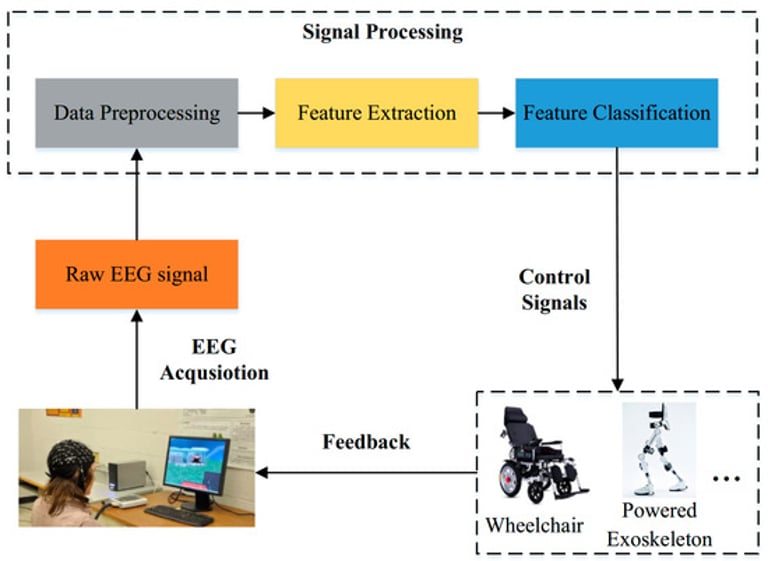
Researchers have developed a new motor imagery classification model called MST-DGCN.
MST-DGCN uses a combination of Convolutional Neural Networks (CNN) and Graph Neural Networks (GNN).
The model addresses individual variability challenges in EEG-based Motor Imagery Brain-Computer Interfaces (MI-BCIs).
It incorporates distinctive feature fusion with adaptive structural LASSO to extract spatial domain features from EEG signals.
This approach improves classification accuracy.
Experiments on real EEG datasets demonstrate the model's effectiveness.
The model showcases potential for advancements in BCI applications and EEG analysis.
Summary based on 2 sources